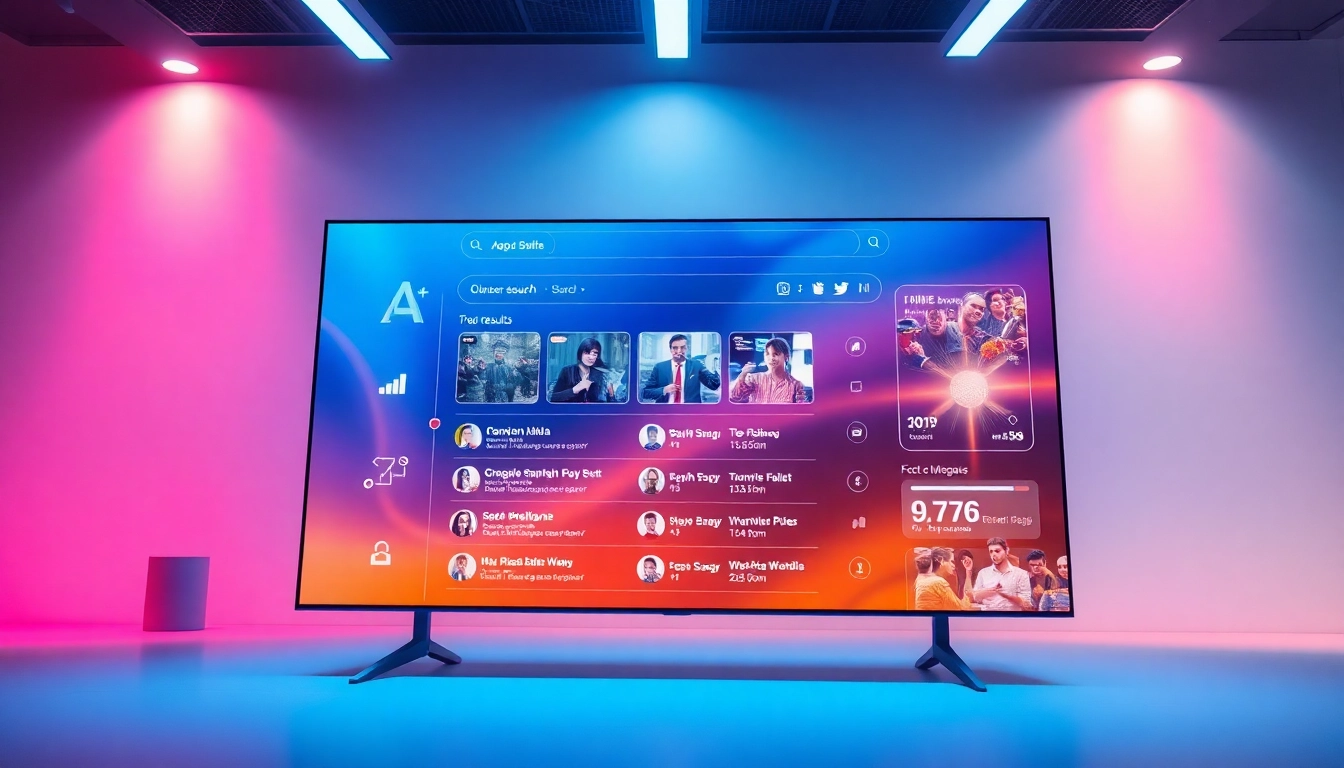
Understanding AI Search: An Overview
With the rapid advancements in technology, the way we search for information is undergoing a profound transformation. Traditional search engines, while effective, often fall short in understanding user intent and providing tailored results. Enter AI search, a revolutionary approach leveraging artificial intelligence to enhance information retrieval. This article will delve into what AI search is, trace its evolution, and explore its numerous benefits, setting the stage for a deeper understanding of its capabilities.
What is AI Search?
AI search refers to the integration of artificial intelligence technologies into search engines to improve the relevance and efficiency of search results. Unlike traditional search engines that rely heavily on keyword matching, AI search engines can understand context, interpret user queries more intelligently, and provide personalized responses. They utilize algorithms based on natural language processing (NLP) and machine learning (ML) to enhance user experiences and deliver information that aligns with user intents.
The Evolution of Search Engines
The history of search engines is marked by continuous innovation. Early search engines like AltaVista relied on simple algorithms that indexed web pages based on keyword frequency. As the web expanded, so did the complexity of search algorithms, leading to the rise of more sophisticated engines like Google, which utilized PageRank to evaluate the importance of web pages based on link structures.
As internet users began to demand more relevant and contextual information, the need for AI-driven search became apparent. The introduction of AI search systems, such as those developed by Google (Gemini) and Microsoft (Copilot), represents a significant leap forward in addressing user expectations for accuracy and contextual relevance.
Benefits of AI in Search Technology
The integration of AI into search technology presents several advantages:
- Improved Relevance: AI algorithms analyze user behavior and preferences to deliver more relevant search results.
- Contextual Understanding: AI can comprehend the context surrounding search queries, allowing for more precise and meaningful results.
- Personalization: By leveraging user data, AI search engines can create personalized experiences that cater to individual needs and preferences.
- Enhanced User Experience: Features such as voice search and conversational interfaces make interacting with search engines more intuitive.
- Continual Learning: Machine learning enables search engines to learn from user interactions, continuously improving their accuracy and efficiency over time.
Leading AI Search Engines: A Comparative Analysis
As AI search technology matures, several platforms emerge as frontrunners in delivering effective search solutions. Understanding the landscape of AI search engines is essential for users and businesses looking to adopt these technologies.
Top AI Search Platforms of 2025
For 2025, several AI search platforms are expected to lead the charge:
- Google Gemini: A product of Google’s innovation, Gemini integrates advanced AI features, including multimodal search capability, which understands various content types like images, text, and video.
- Microsoft Copilot: Part of Microsoft’s suite of productivity tools, Copilot enhances user searches by providing actionable insights and recommendations based on query context.
- ChatGPT Search: Developed by OpenAI, this search engine emphasizes natural language queries, offering conversational answers that give users immediate context and relevant links.
- Bing AI: Microsoft’s Bing has incorporated AI extensively to rival traditional search engines by offering improved search experiences, fueled by real-time user interaction data.
- Perplexity AI: Known for its focus on accuracy, Perplexity uses advanced algorithms to retrieve and synthesize information quickly and reliably.
Features That Set AI Search Apart
AI search engines differ from traditional ones in several key features:
- Multimodal Search: Leveraging multiple input types (text, image, voice), AI search can provide richer results. For instance, users can search by uploading images or asking questions through voice.
- Contextual Query Understanding: These systems can interpret ambiguity and subtleties in language, resulting in more relevant answers.
- Real-Time Data Processing: AI search engines offer real-time processing of queries, ensuring users receive up-to-date information.
- Predictive Search Suggestions: AI analyzes previous queries to suggest potential related searches, improving user navigation and discovery.
- Enhanced Voice Recognition: With advancements in NLP, AI search engines excel in understanding spoken queries, making them accessible to a broader audience.
Case Studies of Successful Implementations
Examining how AI search engines have transformed businesses can provide insight into their potential:
- Case Study 1: Retail Industry – A leading online retailer adopted AI search capabilities, enabling customers to search via voice and image. This resulted in a 30% increase in conversion rates, as users were able to find products more naturally and efficiently.
- Case Study 2: Financial Services – A financial institution utilized AI search to offer personalized financial advice through its customer portal. Users reported higher satisfaction scores, as the system delivered tailored recommendations based on browsing behaviors and prior interactions.
- Case Study 3: Content Platforms – A popular content-sharing platform integrated AI to enhance search functionality. The addition of personalized content suggestions based on user preferences led to a 50% increase in user engagement over six months.
How AI Search Works: Behind the Technology
Understanding the technology behind AI search can help users appreciate its capabilities and potential applications.
Core Algorithms of AI Search
At the heart of AI search are sophisticated algorithms that enable data interpretation and result generation. Key algorithms include:
- Natural Language Processing (NLP): This technology allows AI to understand and interpret human language, analyzing syntax, semantics, and context.
- Machine Learning: AI search engines employ machine learning techniques to analyze user data and improve result relevancy based on patterns observed over time.
- Reinforcement Learning: Some AI search systems use reinforcement learning to learn the best actions through trial and error, optimizing future performance based on user feedback.
Machine Learning and Data Processing Techniques
AI algorithms rely heavily on machine learning for data processing. These techniques can include:
- Supervised Learning: Algorithms are trained on labeled datasets, enabling them to predict outcomes based on input data.
- Unsupervised Learning: This technique helps discover hidden patterns in data without pre-existing labels, often used in clustering similar queries.
- Deep Learning: Utilizing neural networks with many layers, deep learning allows for more complex data analysis, enhancing the ability to understand nuanced language.
User Interaction and Experience Design
The user experience in AI search is significantly improved by focusing on intuitive design principles:
- Conversational Interfaces: Enabling users to interact with the AI through a chat-like format makes searching more relatable.
- Simplified Navigation: AI search reduces the complexity of finding information by utilizing intuitive layouts and smart suggestions.
- Feedback Mechanisms: Many AI search platforms incorporate ways for users to give feedback on results, which further helps optimize search algorithms for future queries.
Challenges in AI Search: Navigating the Landscape
Despite the advancements in AI search, certain challenges must be addressed for ongoing improvement.
Common Limitations of AI Search Engines
Some limitations encountered include:
- Data Dependency: The performance of AI search engines is highly dependent on the quality and quantity of available data. Insufficient or biased data can lead to unreliable results.
- Transparency Issues: The complexity of AI algorithms often leads to a lack of transparency in how search results are generated, making it difficult for users to trust the outcomes.
- Bias in Algorithms: If machine learning models are trained on biased data, this can perpetuate stereotypes and misinformation in search results.
Citation and Information Accuracy Issues
Ensuring accuracy in search results is paramount, yet AI search engines often face challenges in citation integrity:
- Verification Difficulties: AI systems may struggle with verifying the credibility of sources, leading to misinformation being presented as fact.
- Attribution Errors: There are instances where AI fails to appropriately cite the original sources of information, causing ethical and legal concerns.
Future Challenges and Opportunities
As AI search technology evolves, it will face new challenges while also presenting opportunities for growth:
- Adapting to New Use Cases: As demands shift, AI search must adapt to diverse applications across industries.
- Enhancing User Privacy: Striking a balance between personalization and user data privacy will be crucial in maintaining user trust.
- Developing Ethical Standards: There is a growing need for ethical frameworks that govern AI search practices to mitigate biases and misinformation.
The Future of AI Search: Trends and Innovations
The future of AI search is promising, driven by several exciting trends and innovations that will reshape how we access information.
Emerging Technologies Shaping AI Search
The following technologies are set to influence the development of AI search:
- Augmented Reality (AR) and Virtual Reality (VR): These technologies may lead to immersive search experiences, allowing users to interact with information in three dimensions.
- Expanded Multimodal Capabilities: Future platforms will likely incorporate broader data types, enabling richer and more interactive content presentation.
- Blockchain for Credibility: Blockchain technology could be utilized to verify information sources, contributing to enhanced trust in AI-generated search results.
Predictions for AI Search in the Next Decade
Looking ahead, experts predict several outcomes for AI search:
- Widespread Adoption: More businesses and individuals will integrate AI search into their daily operations, enhancing efficiency and productivity.
- Increased Personalization: Expect even more tailored search results driven by advanced data analytics and user profiling.
- Expansion of Voice Search: With voice assistants becoming more capable, voice-activated searches will likely become mainstream for all demographics, including seniors who may prefer this method for ease of access.
Advice for Businesses to Leverage AI Search
Businesses looking to harness AI search technologies can take several proactive steps:
- Invest in Data Quality: Ensure that the data used to train AI models is of high quality and representative of diverse perspectives.
- Focus on User Experience: Prioritize the design and functionality of search interfaces to meet user needs, ensuring the search process is seamless and intuitive.
- Stay Informed about Trends: Keep abreast of emerging technologies and methodologies in AI search to maximize competitive advantages.
- Foster a Culture of Security and Ethics: Develop ethical standards and practices regarding data usage to build trust with users and comply with regulations.