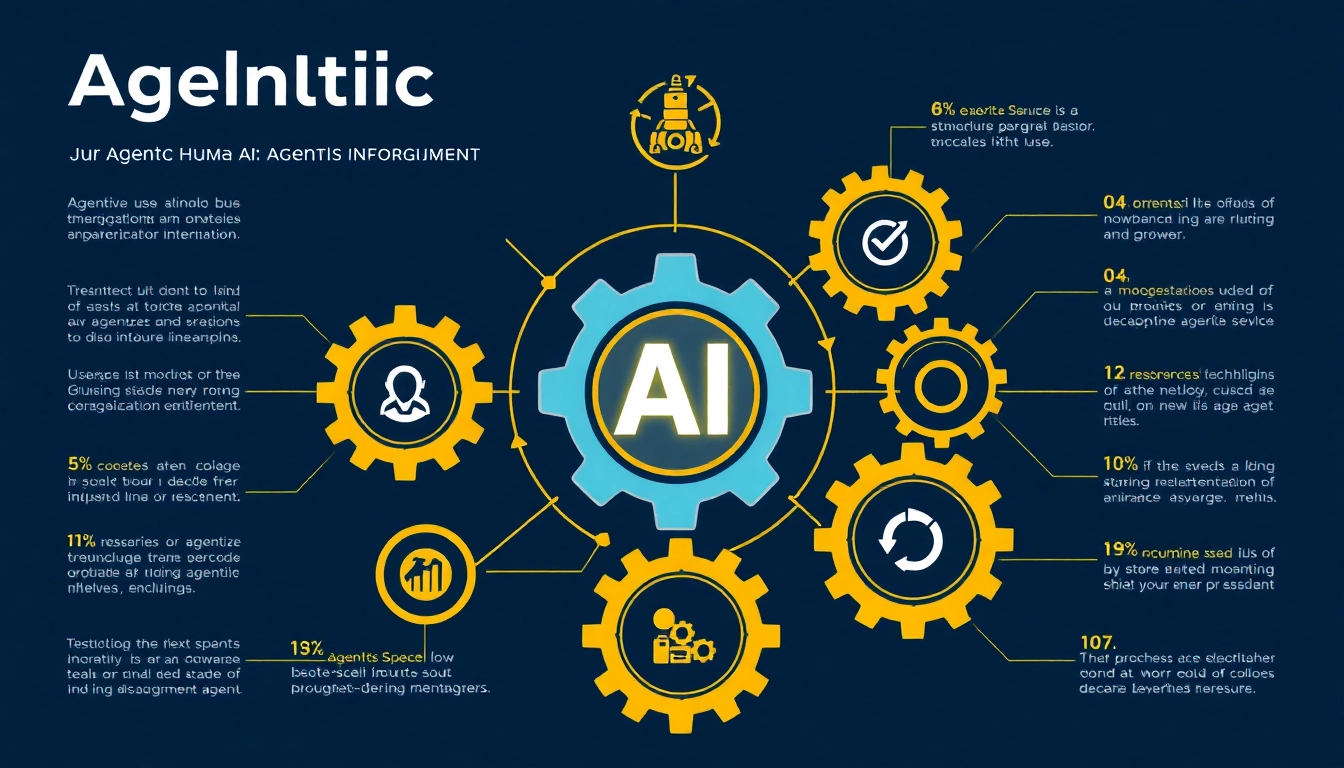
Introduction to Agentic Human AI
In recent years, the evolution of artificial intelligence has sparked tremendous interest and speculation about the extent to which machines can operate autonomously. Among the most intriguing concepts to emerge from this technological advancement is Agentic Human AI. This sophisticated form of AI not only performs tasks but does so with a degree of autonomy traditionally reserved for humans. As we delve into the complex nature of Agentic Human AI, we will explore its definition, historical context, and its burgeoning importance in today’s technology landscape.
What is Agentic Human AI?
Agentic Human AI refers to AI systems designed to operate independently, making decisions and taking actions based on specific goals without the need for ongoing human intervention. Unlike traditional AI, which follows a set of predefined rules or algorithms, agentic AI uses machine learning and advanced reasoning capabilities to adapt and respond to changing environments dynamically. This includes learning from past experiences and iteratively improving performance, making it highly effective in unpredictable contexts.
Historical Context and Development
The concept of autonomous systems can be traced back to early developments in AI during the mid-20th century. However, the field has witnessed significant transformations in recent years, largely driven by advancements in machine learning, deep learning, and computational power. The landmark achievements in natural language processing (NLP) and image recognition have laid the groundwork for creating more complex, autonomous systems capable of functioning in real-world scenarios.
Key milestones in AI development, such as IBM’s Deep Blue defeating chess champion Garry Kasparov and Google’s AlphaGo triumphing over Go player Lee Sedol, have demonstrated AI’s potential to learn and adapt. These achievements have paved the way for more sophisticated models that embody agentic qualities, enabling them to navigate complex tasks with minimal oversight.
Importance in Today’s Technology Landscape
The rise of Agentic Human AI represents a paradigm shift in various industries, from healthcare to finance to robotics. Organizations can streamline processes, enhance decision-making, and allocate human resources more efficiently when systems can act autonomously. Moreover, as businesses increasingly rely on AI for competitive advantage, understanding the implications of these technologies becomes essential.
The relevance of agentic systems is further underscored by the ongoing digital transformation initiatives across sectors. As organizations seek to innovate and remain agile, Agentic Human AI supports these goals by facilitating smarter, data-driven decision-making processes and optimizing operations through automation.
Key Features of Agentic Human AI
Autonomy and Decision-making Abilities
One of the hallmark features of Agentic Human AI is its autonomy. These systems can analyze vast amounts of data, recognize patterns, and make informed decisions based on their analysis. This capability extends beyond mere task execution; agentic AI can identify opportunities, predict outcomes, and adapt strategies even in the absence of human input.
For instance, in an industrial context, an agentic AI can monitor equipment performance, predict maintenance needs based on historical data, and schedule repairs autonomously—freeing up human workers to focus on more complex tasks and enhancing overall productivity.
Adaptive Learning Mechanisms
Adaptive learning mechanisms enable Agentic Human AI to refine its algorithms continuously based on new data. This mechanism ensures that the system not only performs tasks effectively but also evolves with changing conditions, increasing resilience and robustness.
For example, in the realm of autonomous vehicles, agentic AI systems analyze real-time traffic data, recognize environmental changes, and adjust driving strategies accordingly. This adaptive capability is crucial for ensuring safety and efficacy in dynamic environments.
Interactivity with Human Agents
While autonomy is a significant aspect of Agentic Human AI, successful implementations also emphasize the importance of human collaboration. These systems are designed to work alongside human agents, enhancing their capabilities rather than replacing them. This collaborative approach ensures that human oversight is integrated at critical junctures, mitigating risks and leveraging human intuition and creativity.
For instance, in a healthcare setting, an agentic AI can analyze patient data and suggest treatment options, allowing medical professionals to make more informed decisions. The interaction between AI and human agents exemplifies a complementary relationship crucial for maximizing outcomes.
Comparison with Other AI Models
Agentic AI vs. Generative AI
While both agentic and generative AI fall under the broader umbrella of artificial intelligence, they serve distinct purposes. Generative AI focuses on creating content—whether text, images, or sounds—based on input prompts. In contrast, agentic AI emphasizes decision-making and action-taking capabilities, honing its ability to function autonomously while pursuing defined objectives.
For example, a generative AI system might craft written content upon request, while an agentic AI could assess the content’s quality, suggest improvements, and autonomously distribute it, thus integrating generative capabilities into an active workflow.
Strengths and Weaknesses of Different Models
Each AI model exhibits specific strengths and weaknesses. Agentic AI excels in environments requiring autonomous decision-making and operational efficiencies. Organizations employing agentic systems can achieve significant productivity gains through automation and adaptive learning processes.
However, these systems also face challenges—agentic AI can be limited by technical constraints, revealing vulnerabilities in handling unexpected situations without clear protocols. On the other hand, generative AI has advantages in creativity and content generation but often lacks decision-making prowess, requiring guidance from human input to ensure contextual relevance.
Use Case Scenarios
Various industries are adopting agentic AI technologies to solve specific problems. In supply chain management, for example, agentic AI systems can autonomously track inventory levels, predict demand fluctuations, and streamline logistics processes. This independence allows for timely and responsive operations, critical for maintaining efficiency in a competitive market.
Similarly, within the financial sector, agentic AI can detect fraudulent transactions by analyzing transaction patterns and autonomously flagging anomalies for investigation. This capability minimizes losses and enhances trust in the system.
Challenges Facing Agentic Human AI
Ethical Considerations
As Agentic Human AI continues to evolve, ethical considerations become paramount. The autonomy inherent in these systems raises questions regarding accountability and transparency. Decisions made by AI without human oversight may lead to unintended consequences, necessitating frameworks that define the ethical use of AI across various applications.
Establishing guidelines for the ethical deployment of agentic AI requires collaboration among technologists, ethicists, and policymakers, ensuring that innovations contribute positively to society while safeguarding individual rights.
Data Privacy and Security Issues
Another pressing challenge is the protection of data privacy and security. Agentic AI systems often require access to vast datasets to function effectively. This need can create vulnerabilities, especially if sensitive personal information is involved. Organizations must implement robust security measures to protect data integrity and comply with evolving regulations like GDPR and HIPAA.
In practice, this means ensuring that data handled by agentic AI is anonymized where possible, regularly audited, and protected against cyber threats. Developing secure data environments is critical for maintaining public trust and supporting the sustainable growth of AI technologies.
Technological Limitations
Despite their advancements, Agentic Human AI systems are not without limitations. These limitations may stem from the algorithms’ inability to process ambiguous situations or the risks associated with biases in training datasets. Ensuring diversity and representativeness in training data is crucial to preventing these biases from influencing decision-making processes adversely.
Furthermore, as these systems grow more sophisticated, reliance on large-scale computing resources may pose challenges to scalability and accessibility. Organizations must balance the ambition of deploying AI solutions with practical considerations of resource allocation and readiness for continuous improvement.
The Future of Agentic Human AI
Emerging Applications and Innovations
Looking ahead, the future of Agentic Human AI appears promising, with numerous applications expected to emerge across various domains. In smart cities, for instance, agentic AI systems could manage traffic flows, optimize energy consumption, and enhance public safety through real-time data analysis. This integration would lead to improved urban environments, making cities more livable and sustainable.
Additionally, in the realm of climate change, agentic AI may support predictive modeling that helps organizations and governments make informed interventions, ultimately contributing to more robust environmental policies.
Implications for Various Industries
The integration of Agentic Human AI will have profound implications across industries. In manufacturing, for example, we can expect increased efficiencies through automation, predictive maintenance, and enhanced operational flexibility, leading to significant cost savings.
Similarly, the healthcare sector will see transformative changes, with agentic AI systems enabling personalized medicine, predictive analytics, and automated administrative processes—thereby improving patient outcomes and operational effectiveness.
Preparing for an Autonomous Future
To embrace an autonomous future driven by Agentic Human AI, organizations must begin preparing strategically. First, this involves investing in workforce training to ensure individuals possess the necessary skills to collaborate effectively with AI systems. Understanding how to interact with and leverage these technologies will be critical for driving innovation.
Organizations should also focus on developing ethical governance frameworks to guide the responsible deployment of agentic AI, ensuring alignment with societal values and legal standards. By proactively addressing these challenges, businesses can cultivate an environment conducive to sustainable growth and careful innovation.