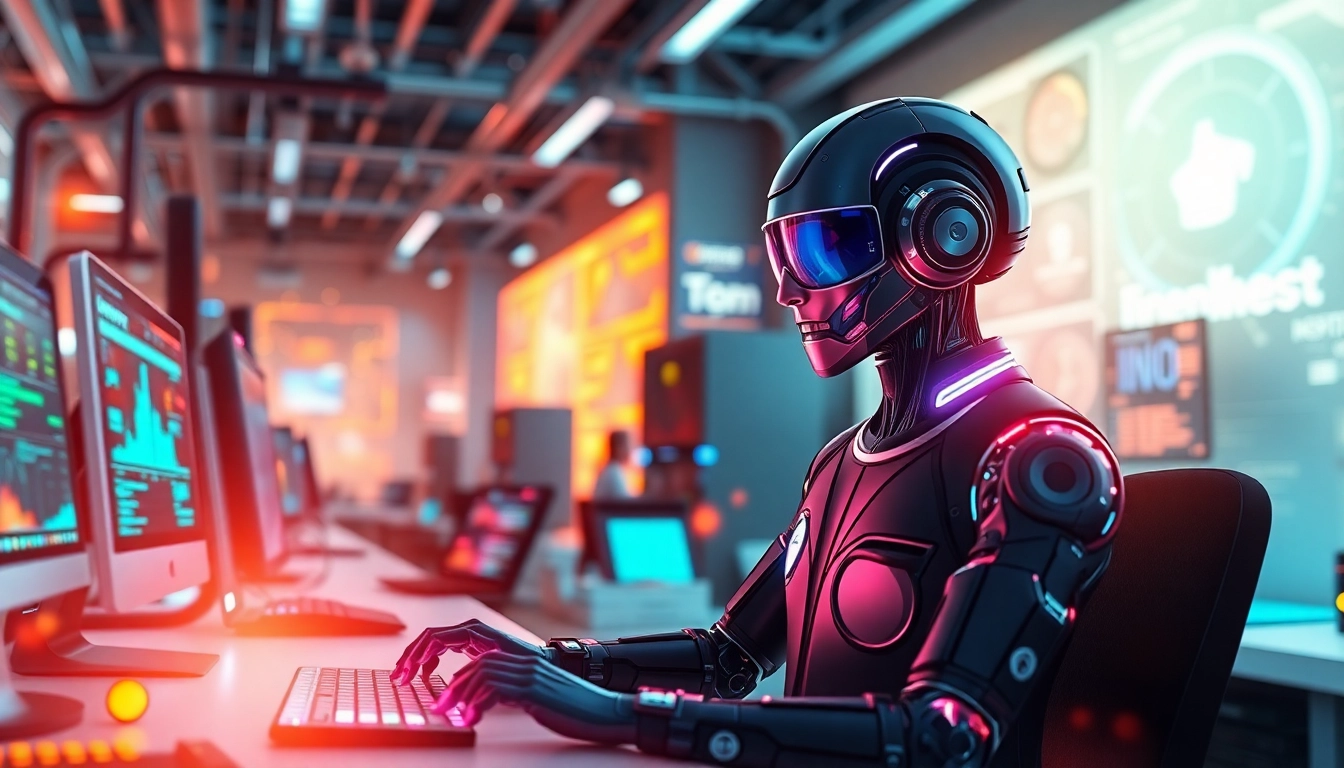
What is Agentic AI?
In recent years, the evolution of artificial intelligence has paved the way for innovative concepts like Agentic AI. This revolutionary technology represents a significant departure from traditional AI paradigms, enabling systems to operate with a higher degree of autonomy. Agentic AI is essentially a class of artificial intelligence that can independently make decisions, perform tasks, and adapt to changing environments, often without the need for continuous human oversight.
Definition and Characteristics
Agentic AI refers to AI systems designed for autonomous operation. These systems utilize advanced algorithms that empower them to not only process information but also act upon it, achieving specific goals. Unlike traditional machine learning models, which might require human intervention at various stages, Agentic AI solutions can evaluate situations, learn from their surroundings, and implement strategies based on real-time data. Key characteristics of Agentic AI include:
- Autonomy: Ability to operate independently, making decisions without human input.
- Goal-oriented: Focused on achieving specific, often complex objectives.
- Real-time adaptability: Capable of adjusting actions based on environmental feedback and changing contexts.
- Problem-solving: Equipped to tackle multi-step problems that arise during task execution.
How Agentic AI Differs from Other AI Types
Understanding the distinction between Agentic AI and other forms of artificial intelligence is crucial for grasping the significance of this technology. Unlike traditional AI—which includes narrow models designed for specific tasks and can often rely on direct human oversight—Agentic AI encompasses a broader scope:
- Narrow AI: These systems excel at individual tasks, such as data categorization or recommendation—all requiring defined parameters and frequently human intervention. Examples include recommendation systems in e-commerce and chatbots.
- Generative AI: Focused on creating content, generative AI systems produce text, images, or music based on user prompts but still require some level of human guidance.
- Agentic AI: Unlike its counterparts, Agentic AI operates with an independence that allows it to pursue complex objectives without needing constant guidance, analyzing new data and adjusting its actions accordingly.
Real-World Applications of Agentic AI
The applications of Agentic AI are extensive, impacting various sectors by simplifying operations and enhancing productivity. Here are some notable implementations:
- Healthcare: Agentic AI systems can autonomously monitor patient health and predict necessary interventions, improving outcomes and healthcare efficiency.
- Finance: In trading and risk assessment, these systems analyze market trends in real-time, making quick decisions that can leverage financial opportunities or mitigate risks.
- Supply Chain Management: Autonomous agents manage stock levels, logistics, and even customer interactions to optimize supply operations without human oversight.
- Manufacturing: Agentic AI can oversee production processes, identifying inefficiencies and adjusting manufacturing techniques autonomously to enhance effectiveness.
- Customer Service: By utilizing chatbots that learn and adapt based on customer interactions, businesses improve responsiveness and satisfaction without the need for continuous human involvement.
Benefits of Implementing Agentic AI
Enhanced Efficiency and Decision-Making
The deployment of Agentic AI can lead to substantial improvements in both efficiency and decision-making capabilities. By automating intricate processes, organizations can minimize human error and increase throughput. Some advantages include:
- Speed: Machine learning algorithms can analyze vast amounts of data and make decisions in real-time, far surpassing human capabilities.
- Consistency: Unlike humans, Agentic AI does not suffer from fatigue or emotional bias, leading to more reliable outcomes.
- Informed Decisions: By continuously learning and adapting, these systems utilize a wealth of historical and current data to inform their actions.
Cost Savings and Resource Optimization
Integrating Agentic AI can significantly impact operational costs. By automating routine tasks, organizations can reallocate human resources to focus on strategic initiatives, thereby:
- Reducing Labor Costs: With AI taking over repetitive tasks, businesses can cut down on staffing expenses.
- Efficient Resource Allocation: AI systems help optimize resource use, leading to reduced waste and costs associated with material and energy consumption.
- Minimized Downtime: Predictive AI can foresee equipment failures, allowing preemptive maintenance that prevents costly interruptions.
Scalability in Various Industries
One of the noteworthy advantages of Agentic AI technologies is their inherent scalability, which can:
- Adapt: Scale operations up or down based on demand or market trends without needing extensive human intervention to recalibrate processes.
- Expand Applications: Easily transferred across different functions or sectors, allowing businesses to leverage AI capabilities in various aspects of their operations.
- Grow with Business Needs: As companies evolve, these systems can be trained to meet new challenges and objectives without losing efficiency.
Challenges and Considerations
Ethical Implications of Autonomous AI
The rise of Agentic AI does not come without concerns, particularly regarding ethics and accountability. As AI systems begin to make decisions traditionally reserved for humans, several ethical questions arise:
- Accountability: In situations where AI takes independent actions that result in negative outcomes, determining accountability can become complicated.
- Bias in Decision-Making: If an AI system is trained on biased data, it may perpetuate or even exacerbate these biases in its autonomous decisions.
- Privacy Concerns: The data required for Agentic AI systems must be collected ethically, respecting users’ privacy and data protection regulations.
Integration with Existing Systems
Incorporating Agentic AI into existing operational frameworks can be challenging, particularly for organizations with entrenched systems and processes. Key considerations include:
- Compatibility: Ensuring that new AI solutions seamlessly integrate with legacy systems can require considerable resources and time.
- Change Management: Employees may resist changes introduced by AI systems. Comprehensive training and engagement are crucial to overcoming these hurdles.
- Cost of Transition: Initial investment costs can be high, leading organizations to carefully assess long-term benefits against short-term expenditures.
Continuous Learning and Adaptation
Agentic AI’s effectiveness relies heavily on its capacity to learn and adapt. Continuous learning presents both opportunities and challenges that organizations must navigate:
- Managing Model Drift: As external conditions change, AI systems can become less effective if not continually updated with new data.
- Resource Intensiveness: Maintaining and improving AI performance can demand significant computational resources and expertise.
- Evaluating Performance: Establishing metrics to assess AI learning outcomes and the quality of decisions taken autonomously is crucial for ongoing success.
Future Prospects of Agentic AI
Trends and Innovations to Watch
The field of Agentic AI is rapidly evolving, with several trends projected to shape its future:
- Increased Interoperability: As more systems embrace Agentic AI, the demand for interoperable solutions that can communicate and work with each other is expected to grow.
- Human-AI Collaboration: Future applications are likely to focus on AI augmenting human decision-making rather than wholly replacing it, fostering better partnerships.
- Greater Accessibility: As technology advances, tools for developing and deploying Agentic AI are becoming more user-friendly, allowing small businesses to harness its power.
Potential Impacts on the Workforce
The rise of Agentic AI brings forth both opportunities and challenges for the workforce. A notable concern includes the potential displacement of jobs forms traditionally filled by humans. However, experts argue that AI will also create new roles that focus on managing and collaborating with autonomous systems:
- Job Transformation: Many roles will evolve, emphasizing skills that complement AI capabilities, such as strategy, creativity, and relationship management.
- Reskilling Requirements: Organizations must invest in retraining their workforce to prepare employees for a future where AI plays a central role in operations.
- New Job Creation: While some jobs may become obsolete, new sectors focused on AI development, management, and ethics will emerge, demanding fresh skills and expertise.
Global Adoption and Investment Trends
Looking ahead, trends in global adoption and investment in Agentic AI are of significant interest. Organizations worldwide are recognizing the potential of this technology, leading to greater levels of funding and research initiatives:
- Increased Investment: A marked increase in venture capital funding toward AI startups indicates a robust belief in the future potential of Agentic AI technologies.
- Global Collaborations: Partnerships between tech firms, academic institutions, and governments are set to fuel innovation and regulatory considerations in the space.
- Policy Development: As adoption scales, governments will be tasked with developing frameworks that address the ethical, legal, and societal implications of Agentic AI deployment.
Getting Started with Agentic AI
Steps for Successful Implementation
For organizations looking to integrate Agentic AI, a strategic approach ensures successful adoption. Here are the fundamental steps to consider:
- Assess Needs: Identify specific problems or challenges that Agentic AI can address within your organization.
- Define Objectives: Establish clear goals for what you aim to achieve with AI implementation.
- Evaluate Readiness: Consider current IT infrastructure, workforce skills, and organizational culture before proceeding.
- Select Appropriate Tools: Choose the right platforms that align with your goals, ensuring they offer the scalability and features required.
- Run Pilot Programs: Implement small-scale projects that allow for testing and iterative learning before a full rollout.
- Review and Adjust: Continuously monitor performance and adapt strategies as necessary based on feedback and outcomes.
Key Tools and Platforms for Development
Several tools and platforms facilitate the development and deployment of Agentic AI solutions. These include:
- TensorFlow: An open-source platform widely used for developing machine learning models, including those used in Agentic AI.
- Pandas: A powerful data manipulation and analysis library in Python, useful for preparing data for AI training.
- PyTorch: Another open-source machine learning library tailored for applications in deep learning, commonly used for building neural networks.
- Google Cloud AI: Provides various AI services for developing AI applications, including tools for natural language processing and computer vision.
Measuring Success and Performance Indicators
Establishing performance metrics is essential for gauging the success of Agentic AI initiatives. Consider the following indicators:
- Operational Efficiency: Measure improvements in process speed and reduction in human error.
- Return on Investment (ROI): Calculate the financial benefits accrued from implementing Agentic AI systems compared to the associated costs.
- Achievement of Objectives: Assess whether the AI systems successfully meet predefined goals and objectives.
- User Satisfaction: For customer-facing applications, evaluating user experience can provide critical insights into effectiveness.