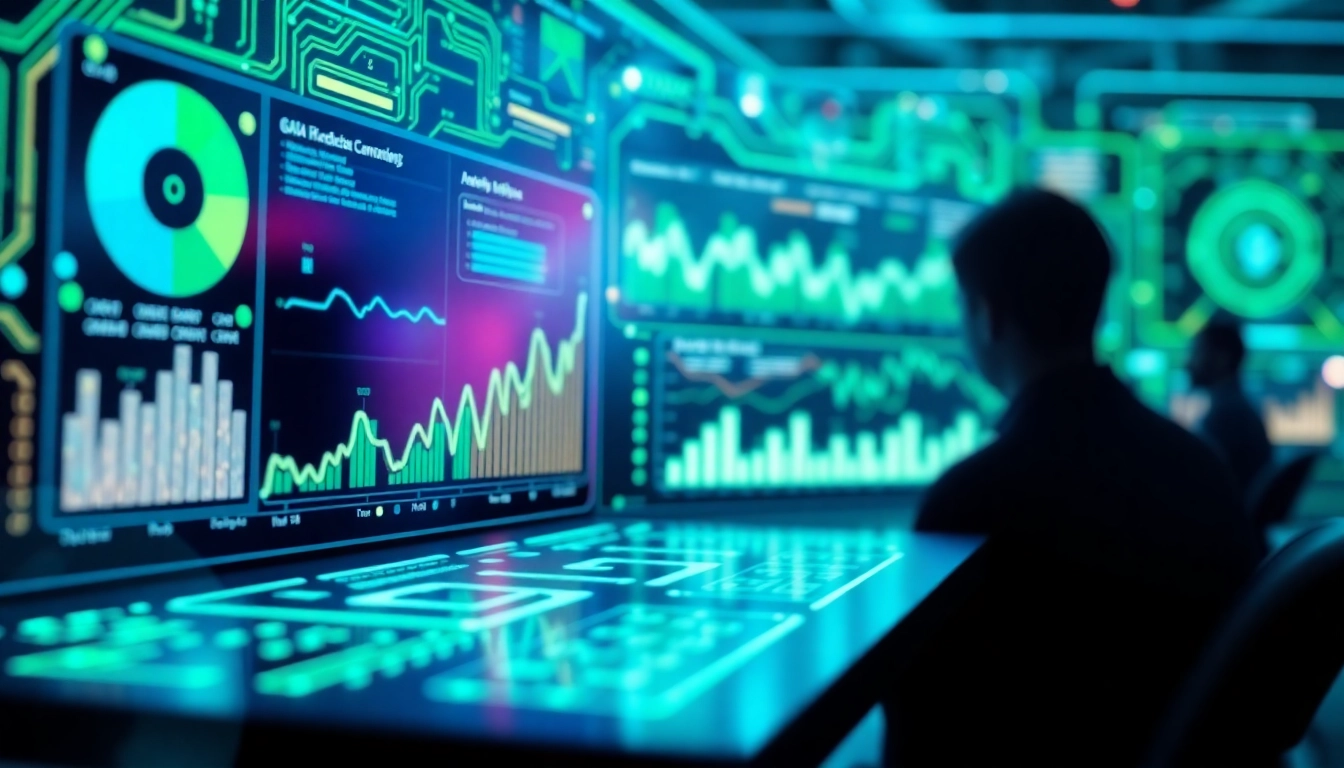
What is Agentic AI?
In the rapidly evolving landscape of artificial intelligence, Agentic AI emerges as a groundbreaking paradigm. Distinct from traditional AI, agentic AI is designed to operate autonomously, enabling systems to make decisions and execute tasks without constant human intervention. This capability positions agentic AI as a transformative force across various industries, paving the way for unprecedented levels of efficiency, adaptability, and problem-solving sophistication.
Definition and Core Principles
Agentic AI refers to systems that have the ability to act independently to achieve specific goals. Unlike other forms of AI that might require detailed instructions for every task, agentic AI incorporates advanced reasoning and planning capabilities. These systems leverage algorithms that allow them to interpret data, assess circumstances, and decide on actions that align with their objectives.
At the core of agentic AI are several principles:
- Autonomy: The capacity to operate without ongoing human input.
- Goal-Oriented Action: The ability to pursue defined outcomes effectively.
- Sandboxing: The use of controlled environments to safely experiment with decision-making processes while learning from their outcomes.
- Adaptability: The skill to adjust to new data or changes in the environment rapidly.
How Agentic AI Differs from Traditional AI
The distinction between agentic AI and traditional AI lies in how tasks are approached and executed. Traditional AI systems often rely heavily on predefined rules and extensive human oversight. In contrast, agentic AI systems function based on learned experiences and can autonomously handle complex scenarios without explicit programming.
For example, while a traditional AI may be programmed to handle customer inquiries using scripted responses, an agentic AI can autonomously adapt its responses based on the specific context of the interaction and learn from user feedback to improve its performance over time, reflecting a more nuanced understanding of human interaction.
Key Characteristics of Agentic AI Systems
Agentic AI systems exhibit several defining characteristics that set them apart:
- Self-Learning: They can improve their performance through experience and interaction with their environment.
- Decision-Making: Capable of evaluating multiple potential actions and selecting the optimal one.
- Context Awareness: Understanding surrounding factors that influence decisions to achieve better outcomes.
- Multi-Step Problem Solving: Ability to break down complex tasks into manageable steps, determining the best path to resolution.
Applications of Agentic AI
The versatility of agentic AI spans across numerous fields, making it applicable in various industries including healthcare, finance, manufacturing, and customer service. Its unique capabilities enable organizations to streamline operations, improve customer engagement, and drive innovation.
Use Cases Across Industries
Here are several notable applications of agentic AI:
- Healthcare: In healthcare, agentic AI systems can assist in diagnosing diseases by analyzing patient data and medical histories, providing recommendations for treatment, or even managing patient scheduling autonomously.
- Finance: Financial institutions utilize agentic AI in fraud detection systems that analyze transaction patterns and identify anomalies, enabling quicker response times to potential threats.
- Manufacturing: Smart factories employ agentic AI for inventory management, logistics, and predictive maintenance, optimizing production lines based on real-time data.
- Customer Service: Chatbots powered by agentic AI can interact with customers to resolve queries, understand their issues, and provide solutions without human oversight.
Impact on Productivity and Efficiency
Implementing agentic AI can lead to significant improvements in productivity and operational efficiency. By automating processes that require minimal human guidance, organizations can reduce labor costs and allocate human resources to more complex tasks that require creative thinking. Studies have shown that companies utilizing agentic AI can increase their operational speed by as much as 30%, resulting in higher customer satisfaction rates and lower operational downtime.
Examples of Successful Implementation
Several companies have successfully integrated agentic AI into their operations:
- NVIDIA: NVIDIA has led the way in leveraging agentic AI for enhancing their computing solutions, employing sophisticated algorithms that allow their systems to solve complex problems autonomously.
- Pfizer: In pharmaceutical research, Pfizer uses agentic AI for drug discovery processes that significantly reduce the time from concept to market.
- IBM: IBM’s Watson employs agentic capabilities in processing large data sets to provide actionable insights for organizations in real-time.
Challenges and Limitations
Despite its promise, the deployment of agentic AI does not come without challenges. As these systems evolve, so do the complexities associated with their implementation and governance.
Current Technical Hurdles
Several technical challenges face organizations seeking to deploy agentic AI, including:
- Integration with Existing Systems: Many businesses encounter difficulties integrating agentic AI into their current infrastructures, often requiring significant changes to legacy systems.
- Data Privacy Concerns: As agentic AI systems collect and analyze vast amounts of personal data, organizations must navigate complex privacy laws and ethical considerations.
- Complex Problem-Solving: While agentic AI excels at multi-step problem-solving, there are still challenges in the accuracy and reliability of outcomes, especially in highly intricate scenarios.
Ethical Considerations in Autonomous Systems
The autonomy of agentic AI systems raises significant ethical questions regarding accountability and decision-making. As these systems take on more responsibilities traditionally held by humans, the implications of errors and biases must be carefully managed. Organizations need to establish governance frameworks that ensure transparency and accountability in decision-making processes.
Future Outlook for Agentic AI
The future of agentic AI is promising, with ongoing advancements in machine learning and neural networks facilitating the development of even more sophisticated systems. As organizations continue to explore the potential of agentic AI, increased collaboration between AI developers and industry experts will be essential to align the technology with strategic business goals and ethical guidelines.
Comparing Agentic AI with Generative AI
Agentic AI and generative AI represent two distinct approaches within the AI landscape. While both serve important functions, their applications and operational frameworks differ significantly.
Functional Differences Explained
The primary distinction lies in their operational focus:
- Agentic AI: Focuses on executing specific tasks and solving problems autonomously.
- Generative AI: Primarily involved in creating new content, such as text, images, or music, based on existing data.
For instance, while agentic AI might manage logistics for a retail store, generative AI could design promotional materials automatically based on marketing preferences.
Current Trends in AI Development
The landscape of AI continues to rapidly evolve, with both agentic and generative AI gaining traction. Notable trends influencing the development of agentic AI include:
- Increased Autonomy: As systems become more sophisticated, they will handle increasingly complex tasks with minimal oversight.
- Collaborative AI: Future agentic AI systems will work alongside humans to enhance productivity and tackle tasks collaboratively.
- Focus on Ethical AI: With growing emphasis on ethical AI usage, organizations are moving towards ensuring that agentic AI systems are transparent and equitable in their operations.
Market Reception and User Acceptance
As agentic AI technologies are implemented within various sectors, user acceptance remains a critical factor. Organizations must invest in training and education to facilitate a smooth transition and build trust among employees. The long-term success of agentic AI will depend on demonstrating value and tangible benefits to end-users.
Steps to Implement Agentic AI in Your Business
Implementing agentic AI requires careful planning and strategic execution. Below are key steps organizations can take to ensure a successful rollout:
Assessing Organizational Needs
Before adopting agentic AI, organizations must assess their specific needs and identify relevant use cases. This involves evaluating current processes, areas where automation could drive efficiency, and pinpointing potential challenges that may arise during implementation.
Integrating Agentic AI Solutions
Integration should prioritize flexibility to incorporate agentic AI into existing systems. This may include utilizing cloud-based solutions that allow for scalability and easier management of data across platforms. Engaging with experienced AI vendors can facilitate a smoother integration process and provide access to ongoing support.
Measuring Performance and Outcomes
After deployment, it’s critical to set clear metrics for evaluating the performance of agentic AI. Organizations should establish key performance indicators (KPIs) to assess the impact of agentic AI on operational efficiency, customer satisfaction, and ROI. Continuous monitoring and iterative improvements based on performance data will refine the system and enhance its effectiveness over time.