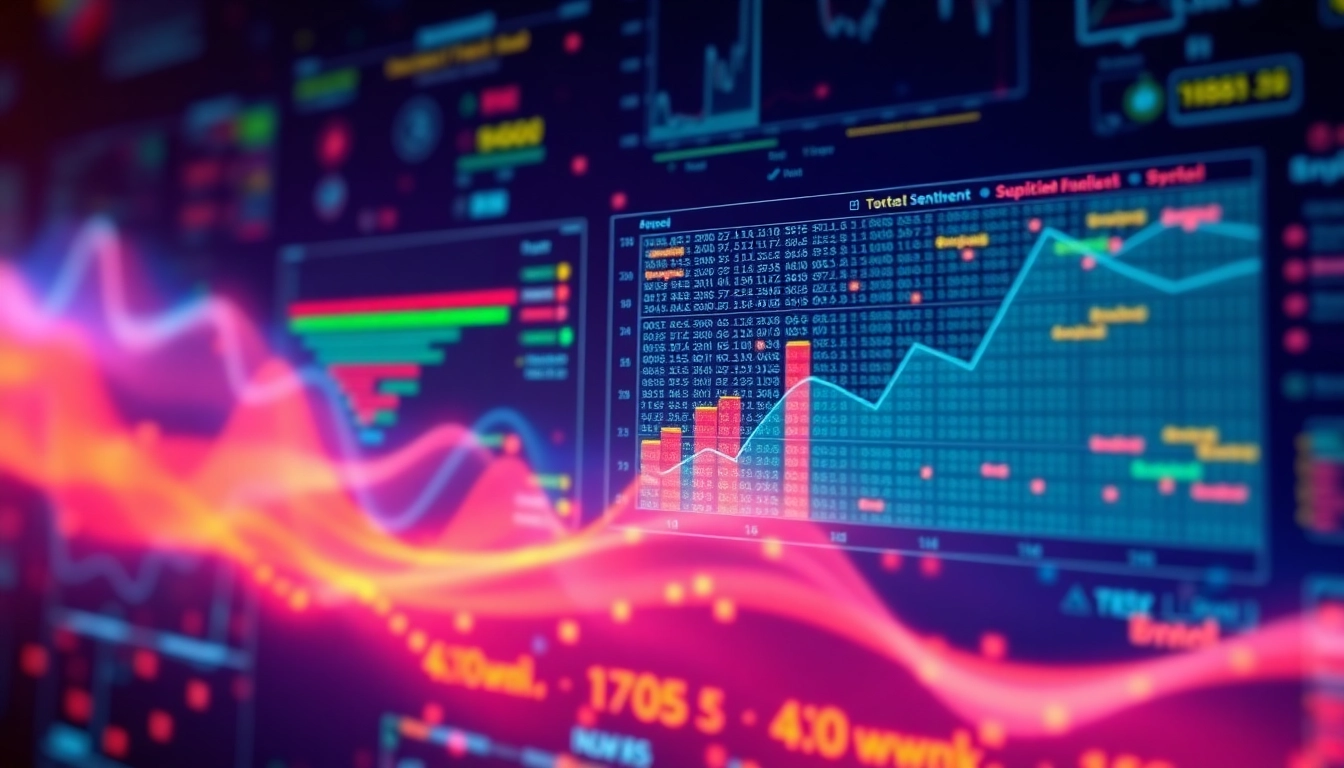
Understanding AI Sentiment Analysis
In today’s data-driven world, understanding customer sentiments has become more crucial than ever. The emergence of AI sentiment analysis tools has transformed how businesses gauge public opinion, enabling them to adapt strategies accordingly. By leveraging advanced algorithms and machine learning techniques, organizations can evaluate the emotional tone behind texts—be it from social media, customer reviews, or survey data. This article delves into the core aspects of AI sentiment analysis, exploring its techniques, applications, benefits, and future prospects.
What is AI Sentiment Analysis?
AI sentiment analysis refers to the computational process of analyzing textual data to determine whether the expressed sentiment is positive, negative, or neutral. This process relies heavily on natural language processing (NLP) and machine learning algorithms to interpret and understand human emotions hidden within textual data. More than merely counting positive or negative words, modern AI sentiment analysis considers context, irony, and varying expressions of sentiments, enhancing its accuracy and breadth.
Key Techniques and Tools
The foundation of AI sentiment analysis rests upon several key techniques and tools that have evolved over the years. Some of the most notable include:
- Natural Language Processing (NLP): NLP techniques are critical to breaking down text data into components that machines can understand. From tokenization to syntactic parsing, these processes help identify the sentiment expressed.
- Machine Learning Algorithms: Algorithms such as Support Vector Machines, Naive Bayes, and deep learning models (like LSTM and transformers) are widely used to classify sentiments based on input data.
- Sentiment Lexicons: These are dictionaries containing words associated with specific sentiments. Ambiguity in language can be addressed by mapping words to their associated feelings.
- Data Annotation: Training datasets for sentiment analysis models require extensive annotation, where texts are labeled with corresponding sentiments to improve algorithm learning.
- Prebuilt Sentiment Analysis Models: Services offered by platforms like IBM Watson or Google Cloud provide out-of-the-box sentiment analysis tools, allowing for seamless integration into existing applications.
Applications Across Industries
AI sentiment analysis has found applications across numerous industries, revolutionizing how businesses perceive customer interactions:
- Marketing: Brands leverage sentiment analysis to monitor public reactions to their campaigns, adjust marketing strategies in real time, and better target their audience.
- Customer Service: By analyzing feedback and support responses, companies can improve services and enhance customer satisfaction, addressing negative sentiments proactively.
- Product Development: Insights derived from sentiment analysis guide product teams on customer preferences and dislikes, enabling iterative development that resonates with users.
- Politics and Social Research: Analyzing social media sentiments regarding political figures or policies helps gauge public opinion and voter sentiment dynamically.
- Healthcare: Sentiment analysis interprets patient feedback and reviews, guiding hospitals and clinics in providing better care and service.
Benefits of AI Sentiment Analysis
Enhancing Customer Experience
Understanding customer sentiment enhances the overall customer experience by enabling companies to react promptly to feedback. By analyzing sentiments across various touchpoints, businesses can tailor interactions to match customer expectations, ensuring satisfaction and loyalty.
Driving Marketing Strategies
Marketing departments utilize sentiment analysis to gauge the effectiveness of their campaigns. By analyzing sentiments about brand messaging, promotions, and overall brand image, marketers can make informed decisions that align more closely with consumer preferences.
Improving Product Development
Product teams benefit significantly from sentiment analysis by accessing real-time feedback on features, issues, and overall user satisfaction. This insight allows teams to refine products based on accurate customer sentiment rather than assumptions, leading to superior outcomes and user satisfaction.
Implementing AI Sentiment Analysis
Choosing the Right Tools
Selecting the appropriate tools for sentiment analysis is crucial. Businesses must consider factors such as accuracy, ease of integration, scalability, and support. Popular tools include:
- IBM Watson Natural Language Understanding: Offers powerful analytical capabilities with customizable features.
- Google Cloud Natural Language API: Provides easy access to sentiment analysis without requiring in-depth technical knowledge.
- Microsoft’s Text Analytics: A part of Azure’s suite, it allows for comprehensive sentiment evaluation.
- Social media insights tools: These tools specifically analyze sentiments expressed in social media platforms.
Data Collection Methods
Data for sentiment analysis can be gathered from various sources, including:
- Social Media: Platforms like Twitter, Facebook, and Instagram are rich sources of raw sentiment data.
- Customer Reviews: Aggregating reviews from sites like Yelp or Amazon can yield critical insights into product satisfaction.
- Surveys and Feedback Forms: Direct customer feedback provides structured data that can be analyzed for sentiment.
- Web Analytics: Monitoring user interactions across websites can help identify sentiment through behavior patterns.
Integrating with Existing Systems
A successful implementation of AI sentiment analysis requires seamless integration with existing systems. This often involves:
- API Integrations: Utilizing APIs from various tools ensures that sentiment data is directly embedded into workflows or dashboards.
- CRM Systems: Enriching customer relationship management tools with sentiment data helps customer service teams provide tailored responses.
- Visualization Platforms: Using analytics platforms like Tableau or Power BI to visualize sentiment trends effectively enhances data interpretation.
Measuring the Impact of AI Sentiment Analysis
Key Performance Indicators
Measuring the impact of sentiment analysis initiatives involves defining key performance indicators (KPIs). This could include:
- Sentiment Score: A quantifiable score that summarizes the overall sentiment identified in the data.
- Customer Engagement Rates: Monitoring engagement levels before and after implementing sentiment analysis can show its effectiveness.
- Response Time: Measuring how quickly customer service can address negative sentiments reveals operational efficiency.
- Conversion Rates: Tracking changes in sales or sign-ups after implementing targeted sentiment-driven marketing strategies can reflect the success of these initiatives.
Case Studies and Success Stories
Many organizations have successfully harnessed AI sentiment analysis to drive results. For instance:
- A major retail chain used sentiment analysis on social media feedback, which led to a 15% improvement in customer satisfaction scores after they adjusted their product offerings.
- A tech startup employed sentiments from customer feedback to prioritize features in their application, resulting in a 30% increase in user engagement after the launch of new functionalities.
Continuous Improvement Strategies
In order to maintain a competitive edge, businesses must continuously analyze and update their sentiment analysis practices. Strategies include:
- Regular Training: Continuously retraining algorithms on new data ensures they remain accurate over time.
- User Feedback Loops: Creating mechanisms where users can provide ongoing feedback on sentiment accuracy will enhance model performance.
- Adaptation to Language Changes: As language evolves and new terms emerge, frequent updates to lexicons and models are essential to reflect these changes.
The Future of AI Sentiment Analysis
Evolving Technologies and Trends
The future of AI sentiment analysis holds numerous evolving technologies and trends:
- Generative AI: This technology can assist in understanding sentiment nuances and providing tailored responses based on emotional context.
- Deep Learning Enhancements: Ongoing advancements in deep learning models promise even better accuracy in deciphering sentiments from complex data.
- Multimodal Sentiment Analysis: The combination of text, voice tone, and visual data is anticipated to refine sentiment analysis further, providing richer insights.
Challenges and Considerations
Despite its advantages, businesses face challenges while implementing sentiment analysis:
- Data Privacy: Compliance with data protection regulations such as GDPR is vital when collecting and processing customer data.
- Ambiguity in Language: Sarcasm and context can lead to misinterpretations, necessitating ongoing enhancements to NLP algorithms.
- Dependence on Quality Data: The success of sentiment analysis is contingent on the quality of the data collected, demanding rigorous data cleansing processes.
Preparing for the Future
To successfully navigate the future of AI sentiment analysis, businesses must:
- Invest in Training: Building in-house talent and upskilling current employees can lead to more informed decision-making regarding sentiment analysis.
- Stay Informed: Keeping abreast of new trends and technology in AI will help organizations make proactive adjustments to their strategies.
- Foster a Culture of Data-Driven Decision Making: Encouraging teams to rely on sentiment analysis insights can lead to better business outcomes.